Whenever one is concerned with rare events, events with small probability of occurrence, the Poisson distribution shows up in a natural way. - Falk, Hülser, Reiss
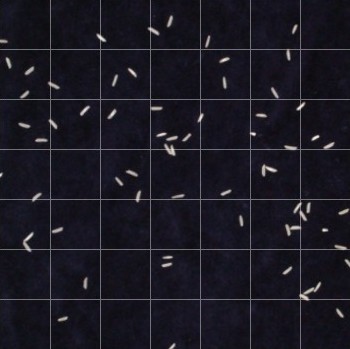
Lets spread some rice corns on a table, each cell represents a person.
Do we expect equal wealth for everyone, given that all have the same virtue and skill?
The Poisson distribution tells us 16 persons get nothing, the probability that you are one of them is 26%.
Over fifty percent get zero or one corn - means they are poor.
Thirtyfour percent are "two or tree corn" people, forming a middle class .
Over fifty percent get zero or one corn - means they are poor.
Thirtyfour percent are "two or tree corn" people, forming a middle class .
A person on the sunny side of life get four and two very lucky ones get even 5 corns, They are the rich five percent of society
#corn | #people | P(X=corn) | |
0 | 16 | 26% | |
1 | 14 | 35% | |
2 | 10 | 23% | |
3 | 6 | 11% | |
4 | 1 | 4% | |
5 | 2 | 1% | upper-crust |
Random distribution of wealth leads to substancial inequality. Some people just make it, while most struggle. Is this the secret formula of "the american dream"?